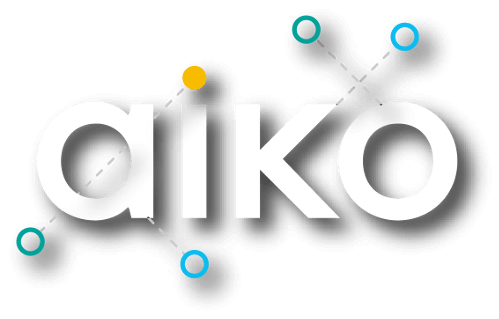
Authors: Gabriele Giordana, AIKO.
The upcoming next generation of satellites will be characterized by a higher level of operative flexibility and automation both from the platform management and payload configuration standpoints. Additionally, augmented connectivity in terms of communication capabilities will result in an unprecedented integration of satellite services with terrestrial deployments.
This scenario leads to an increase in complexity in fulfilling the users’ requirements which is no longer an achievable task for a payload engineer due to the real-time constraints and the number of possibilities; Artificial Intelligence and Machine Learning (AI/ML) algorithms appear to support and enhance the operator decisions
Through the use of AI, it is possible to integrate real-time analytics into space operations, processing current and historical data to prescribe actions in response to any mission and business moment and other events. This combination allows the operators to have frictionless cycle time to derive continuous value and information from all mission, platform, and payload data. It’s a modern data-driven approach to analytics that allows us to quickly get to all the relevant data and accelerate the analysis, which is needed, no matter how off the beaten track it is, no matter how many data sources there are, or how vast the volumes. It’s about letting the system automate the entire analysis and decision process so it’s continuous and seamless.
Information derived from real-time analytics can be used to identify anomalies and scenario changes as they occur. Rather than being reactive, streaming analytics will let to take immediate corrective actions or seize opportunities that otherwise might have been missed.
Operative use cases that are particularly effective combine both the real-time analytics of streaming data and the analysis of historical data.
During the operational phase, a large amount of data flows from the orbiting platforms towards the ground segment. Different types of data can be exchanged, starting from housekeeping and telemetry data up to the data generated by the payload that contains the actual business value of the system. In the case of a system designed to provide a service (telecom, data relays, etc.), the efficiency in maintaining and operating the system represents the added value for the company.
The ability to store and analyze this enormous amount of data in a continuous way is therefore fundamental; then being able to connect this information flow to the decision-making pipeline autonomously represents a distinctive business value for the company that operates the system.
The adoption of AI-based and ML-based algorithms and models could provide augmented functionalities in the context of Ground Analytics, enabling state-of-the-art performances in the context of data management and retrieval, historical and real-time data analysis, optimal network management, operational and business-oriented recommendation system, strategic mission (long-term planning), and finally real-time payload reconfiguration.
The various computational modules implemented in the mission control centre must be perfectly integrated with the edge devices and both segments of the architecture are completely complementary and possibly functionally redundant. The actual optimal configuration is given by the needs of the specific use-cases.
However, the benefits of having a combination of real-time (closer to the device) and more in-depth analysis (ground segment) that can maximize the return and value of the overall distributed architecture remain evident.
At present, even small satellites can be equipped with high computational capabilities thanks to the integration of real edge computers, including commercial ones, capable of running real, modern operating systems, usually based upon the Linux kernel. This means that edge computers can effortlessly multitask, managing multiple applications simultaneously, supporting a wide range of popular networking protocols, and so on. In general, edge computers today can run almost anything that a server computer in a data centre can run. This enables space companies to move appropriate types of application code out of their data centres and into the operational environment.
Edge computers often run multiple applications simultaneously. The edge computers’ modern operating systems enable shared access to hardware resources. Their powerful hardware can perform extremely complex tasks, like machine inferencing. They can analyse data locally and therefore send lower volume, higher value data to the data centre. They also often enable multiple tenants to run applications simultaneously on the same hardware.
In general, edge computing allows to reduce network costs, avoids bandwidth constraints, reduces transmission delays, limits service errors, and better control of sensitive data transfers; moreover, in the context of autonomous platforms, edge computing allows for more reactive and proactive devices, capable of making operational decisions in an increasingly generalized mission environment.
This design pattern will represent the future of data-driven space operations in the upcoming years. The ATRIA project fits perfectly in this line of optimization of the management of space missions, with the idea of providing a high-performing service capable of generating greater added value than in the past.