In phase 1 of the project, two different use cases were tackled by the partners responsible for AI development, AIKO and CTTC. AIKO provided a model for each of them, trying to build effective solutions for telecommunication operators on the specific matters of signal attenuation and traffic congestion.
Use case 1: prediction of rainfall on gateways locations
For this use case, AIKO aimed to develop a DL model capable of providing a prediction about the rainfall rate in the teleport location. The Rainfall has a great impact on the attenuation of the signal and having a precise position on the teleport location can provide useful insights for the decision-making process.
The task of rainfall prediction with deep learning is known in literature as Nowcasting. Nowcasting is a type of deep learning that uses time-series data to make short-term predictions. It uses data from the past and present to make predictions about the near future. Nowcasting can be used for a variety of applications, such as forecasting weather. The nowcasting working flow can also be applied to many other different fields which involve time-series data, such as economy trends.
Convolutional neural networks (CNNs) are used for nowcasting because they are well suited for detecting spatial patterns in data. CNNs are particularly effective at recognizing patterns in images taken from satellite data, which can be used to identify weather patterns and provide more accurate forecasts.
The model is trained considering radar maps that provide a measurement of the rainfall. The DL model takes as input a time series of radar maps regarding the past detected rainfall. The prediction corresponds instead to a map which forecasts the probability of different levels of precipitation 1 hour in the future.
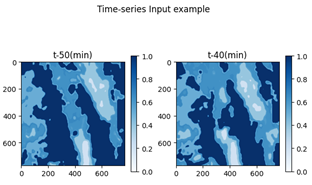
The accuracy of the rainfall prediction is strictly associated with the quality and the cardinality of the involved dataset. In fact, meteorology forecast is not straightforward and the conditions that lead to precipitations can be very different over the same locations also considering the same time period. The results obtained by the model have been positively evaluated, and promising margins for improvement are already envisioned: more data relating to less represented classes and conditions could be collected in order to balance the performance of the model and better generalize; in addition, since precipitation is influenced by several facts, performing a data fusion with other kind of data or weather maps can be a good strategy to further improve the results.
Use case 2: prediction of traffic and beam congestion
Use Case 2 algorithm by AIKO was focused on the prediction of traffic requests on the different beams of the telecommunication infrastructure, in order to provide a forecast of the behavior of traffic load and to warn the operator of potential congestions in the near future.
The task was tackled by adopting a statistical approach modeling a time series as a stochastic process whose every value shows a correlation with the precedent values assumed by the series, with the further addition of gaussian noise.
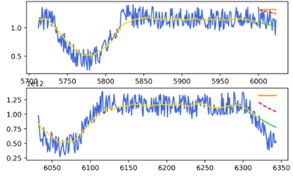
The obtained results showed a significant performance of the algorithm on both synthetically generated datasets and real-time series coming from the operational scenario envisioned for the application.
The trend of traffic load is generally well captured, and the prediction of peaks contains most of the oscillations from the trend. This represents valuable information for the user; the application does not tackle the much more difficult task of predicting every small oscillation in the traffic, but provides an overall evolution of the situation and enough information to foresee potential heavy loads in the near future.
In any case, lower performance is detected during abrupt changes in the traffic trend; the algorithm is capable of forecasting them, but its response is looser than the real trend inversion. Further improvements could be made by tuning the algorithm parameters after a campaign of tests in the operational scenario; this will allow to assess the performance in different cases, and the feedback of users will be a key lead to understanding where the model is suitable and where enhancements are possible.